Financial Institutions Can Put Artificial Intelligence to Much Better Use
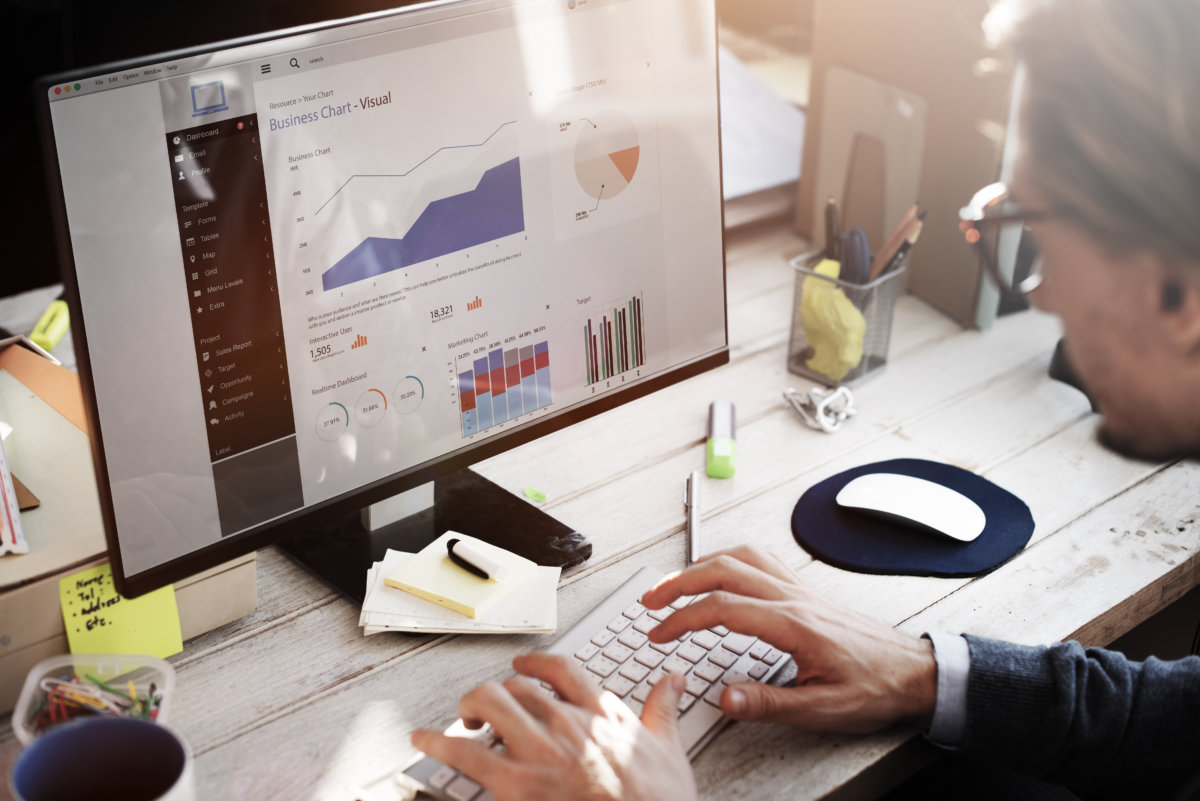
I’ll start with a potentially controversial statement. Banks are misallocating their investment in artificial intelligence and predictive analytics by putting it into consumer- facing chatbots, rather than using it internally to empower their staff to understand and better serve the customer. Most customers don’t like speaking with bots and usually call their bank when they have an issue that requires processing that’s beyond what artificial intelligence can currently offer. In fact, AI’s reputation has been damaged virtually beyond recovery by the endless loop most customers encounter when they call the bank, not able to get to where they want to go. Moreover, you don’t see pictures of chatbots pinned up in banks with “Employee of the Month” emblazoned across the bottom. Nor was any new business won on the strength of a chatbot’s performance. Finally, customers don’t stay with banks because they developed a great working relationship with a chatbot. Truth of the matter, chat hasn’t reached the level where it’s consistently reliable for addressing the customer concerns that rise to the level of making a call to a financial institution. All that said, artificial intelligence is a highly powerful tool.
How it’s being used is simply being misallocated. So the question becomes, is there a way banks can use it to enhance human engagement with clients? The answer is, “Yes.” Although banks and other financial institutions are in a completely different line of business than, say, a luxury retailer or car dealership, what they have in common is that critical need to engage customers at various points in a given transaction. This applies to banks and other financial institutions at least as much as it applies to other businesses. Reaching out to, connecting with and maintaining relationships with customers, and doing it well, is a key consideration. Done well, banks have a better chance of securing a higher lifetime value from their clients when they get it right. And it’s much harder for bankers or advisers to know about the hundreds of products that are available to them; far more so than, say, a car salesman at a dealership, or an associate in the dress department at Saks. AI’s best use is providing them — the customer- facing bank advisers — with the tools to have the right information for the right client, so they can spend more time on the customer relationship.
There are ways in which the power of predictive analytics can be brought to bear immediately, creating a more substantial and recognizable benefit for both financial services providers and their customers. A knowledge-driven approach to cross-selling and upselling is one such strategy. There’s a vast range of training, tools and processes that can positively influence engagement efforts. But predictive analytics can push these initiatives into a much higher
gear, providing a uniquely powerful impact when it comes to solidifying those all- important bonds with customers. Through better analysis and use of data that’s already available to most financial institutions in petabytes, it’s possible to learn more about customers, and consequently offer them more relevant service, support and product options. The right, internal approach to applying predictive analytics, therefore, results in benefits for both customers and the financial services providers they work with — a true win-win situation. Historically, banks — especially large ones — tend to lean more toward conservative, careful approaches to new strategies and technology than quick movement and adoption. Given the mound of compliance mandates that govern their every engagement, this is understandable. But it but can be a significant drawback. This is where predictive analytics can sharpen their game. Many institutions have demonstrated a resistance to adopting this specific tool, or have used it in a very limited way. But they’re missing out on the benefits. And understanding the inherent pitfalls in predictive analytics is key to achieving success in deploying it.
How Financial Institutions Can Effectively Deploy Predictive Analytics
It’s a given that cross-selling and upselling help create more lifetime value from customers. But finding strong connections between products and clients is still a complicated process; particularly when you have to juggle moving parts, such as customer credit scores, income, credit utilization, and the like. Figuring out what products you can sell to whom, and predicting what those outcomes will be, constitutes a successful cross-sell. When done correctly and ethically, cross-selling can ultimately strengthen the customer relationship into a lifetime value — read, profitability — for the bank. This is because they’re able to match a product that was needed with a demand that they’ve identified. It’s 20/20 hindsight, but we all know about the debacle of Wells Fargo’s unethical cross- selling and upselling, and how much trouble it got into as a result. With upselling, predictive analytics can really make a difference in the campaign to upsell. And unlike the Wells Fargo situation, this approach is sustainable. Looking through vast amounts of consumer data can help banks to understand how relationships have historically evolved between the bank and its consumer over time. On the consumer side, the spotlight is on how their data is being used. Only by robust analysis of customer behavior — ideally where multiple products are being offered — can banks regain their customers’ trust that their data is being used to benefit them.
Predictive analytics platforms can conduct this type of analysis, leaning on demographic information, as well as purchasing and financial data that institutions already have from past customer activity. All in real-time. Such an analysis would be prohibitive in terms of time, were trained experts to do the crunching. The predictive analytics tool can then offer sharply defined, personalized, relevant recommendations for staff members to share, while they continue to provide the critical human element in the cross-selling and upselling processes. Where does this data come from? The sheer volume of payments data that banks gather, whether credit card, utilities, rent or many more — can inform what financial product the customer might be looking for and can afford, creating a sharper, more relevant offering. And that’s where artificial intelligence and predictive analytics can play a role that helps bankers sharpen their game and engage more successfully with their customers, without throwing them on the mercy of the bots. Incidentally, it also proves the notion that artificial intelligence is less about displacing humans and more about helping them perform higher- value work.
Securing profitable customers — back to the lifetime value concept — is job No. 1 for banks, whether small or large. Successfully cross-selling — truly matching a product with an identified need — goes a long way to strengthen that customer relationship. The current financial services landscape is ripe for improvement through the use of predictive analytics. Many institutions are already using advanced analytics, tied to marketing and basic interactions — but few have developed strong processes that focus on understanding customer habits and preferences. From there, they can use predictive tools to become more relevant, valuable — and humanly available — to their clients. The institutions that manage to do so will have an advantage in building stronger, longer-lasting relationships and will enjoy the increased value that comes from them.