Improve the FATE of Your Organization’s AI Models
This is an AArete Digital & Technology insight
Artificial intelligence (AI) is transforming how leaders manage their organizations, but the immensely powerful technology comes with one glaring weakness: biases in data that could seep into AI models which unintentionally cause irreparable reputational and financial harm to an organization. Leaders can mitigate bias risk by implementing proven testing techniques to improve the fairness, accuracy, transparency, and equity (FATE) of the output generated by AI.
AI use has grown exponentially over the past year and with it a greater awareness of its impact on society. The terms “transparent AI,” “equitable AI,” and “ethical AI” represent various aspects and ideals within the field of AI development. These terms are often used interchangeably, but they all mean one thing: Responsible AI takes a human-centered approach to minimize bias in output or content generated from the vast array of data in AI models.
Concerns about bias risk have certainly not prevented a surge of investment capital to launch companies building the next generation of AI models. Venture capital firms poured $19.5 billion into AI start-ups and early-stage businesses through the end of September, according to market researcher Pitchfork. That is more than twice the amount invested in the previous two years ($3.1 billion in 2022 and $6.2 billion in 2021).
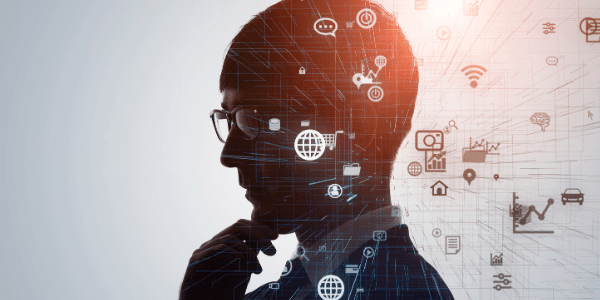
Those investors see the positive impact that AI and generative AI tools like ChatGPT can have on improved operational performance and better customer service. Financial services companies, for example, are leveraging AI models to enhance the customer banking experience. In healthcare, hospitals are using technology with patients to prevent, manage, and reverse complex diseases and chronic conditions.
Bias in AI, however, cannot be ignored because biased data can lead to potentially severe negative outcomes for an organization. Researchers at the Massachusetts Institute of Technology found a wide range of biases in generative AI and large language models that led to gender, racial/ethnicity, and socio-economic stereotyping.
Here is the bad news that leaders must recognize about AI: It is impossible to avoid bias in data because the principal source of bias is the data itself. No data source can be completely free of implicit biases (cultural, gender, racial, socio-economic, political, or religious). Compounding the challenge is that bias can be potentially amplified due to the algorithms that analyze and produce AI-generated output. There is a direct cause and effect. Biased data leads directly to undesired output.
Here is the good news: It is possible to use time-tested, proven techniques to test and adjust for bias and enhance the FATE of your AI.
Types of Bias Risk in AI
- Data selection: If you are building a predictive model and your data is built using only a single source, your AI models might not generalize well to a broader population.
- Unbalanced classes: If the data classes you are trying to predict are not represented equally in your dataset, the model may become biased toward the majority class.
- Outliers: If outliers are simply removed without understanding their cause, it might result in bias.
- Missing data: If data is “missing not at random” (MNAR), simply removing these instances or imputing them can lead to biased results.
- Labeling: Bias can be introduced if the labels in the data are subjective or contain human error.
Bias Testing Techniques
Data scientists typically employ five steps when building an AI model: EDA (exploratory data analysis), model training, testing, validating, and deployment. We believe many organizations are missing another crucial step – quality assurance testing – that can mitigate bias before the AI model is deployed.
Quality assurance testing a model’s functionality – before it is released for organizational use – provides programmers, designers, and developers an opportunity to review an AI model’s limitations and capabilities, identify biases, and then fine-tune the application to achieve the desired results. We recommend organizations focus on the following statistical analysis tools to measure and quantify bias:
- Disparate impact: Assesses if the data unintentionally and disproportionately affects different groups of people.
- Statistical parity: Evaluates if groups receive equitable treatment.
- Equality of opportunity: Determines if all individuals have equal access to an organization’s resources.
- Individual fairness: Analyzes if individuals with similar characteristics are treated fairly.
- Counterfactual fairness: Examines if individuals would be treated fairly if, for example, the person belonged to a different demographic group.
- Fairness through awareness: Calculates if similar people receive similar treatment using a defined metric.
How to Adjust for Bias
Once the underlying data and results have been reviewed, it is imperative that your AI development team revisit the primary forms of bias and use these techniques to “retrain” the model to deliver the desired output:
- Selection bias: Strive to collect a representative sample of the population. If this is not feasible, consider techniques such as reweighting or matching to correct for bias.
- Unbalanced classes: Use techniques such as oversampling the minority class, undersampling the majority class, or data generation methods such as the synthetic minority over-sampling technique (SMOTE).
- Outliers: Use robust methods for outlier detection and consider the context before deciding how to handle them.
- Missing data: Use appropriate imputation methods considering the nature of the missing data.
- Labeling: Conduct multiple label reviews – or aggregation of multiple annotations – and provide clear guidelines so labelers can reduce subjectivity.
What it All Means
AI will revolutionize how organizations manage their operations. Most organizations, however, do not have the necessary in-house capability required to launch an AI model. As a leading global management and technology consulting firm, AArete has the extensive industry knowledge and technical expertise to build, test, and deploy AI technology for your organization. Our digital and technology experts apply a holistic, multi-disciplinary approach to mitigate bias risk and provide ongoing support to provide continuous improvement for your AI model.
If you are eager to improve the FATE of your organization’s AI, contact us today. We are ready to help.
Learn more about AArete’s Artificial Intelligence (AI) solutions